Overview: Crime Landscape
The dashboard reveals that 76.42% of total crime incidents are property-related, making it a key focus for city planners and law enforcement.

The overall trend from 2017 to 2022 shows recurring seasonal patterns that can inform proactive community safety strategies.

Geographic Focus: Downtown Commercial
Neighborhood analysis highlights Downtown Commercial as the highest crime area, with 20,485 property crimes, supported by heatmap visualizations tied to Seattle’s Micro Community Policing Plans (MCPPs).

Insight 1: Assumptions About Property Crime Type
HYPOTHESIS 1: Burglary would be the most common property crime.
Reality: Data shows larceny-theft is most prevalent, proving the value of data-driven decisions over assumptions.
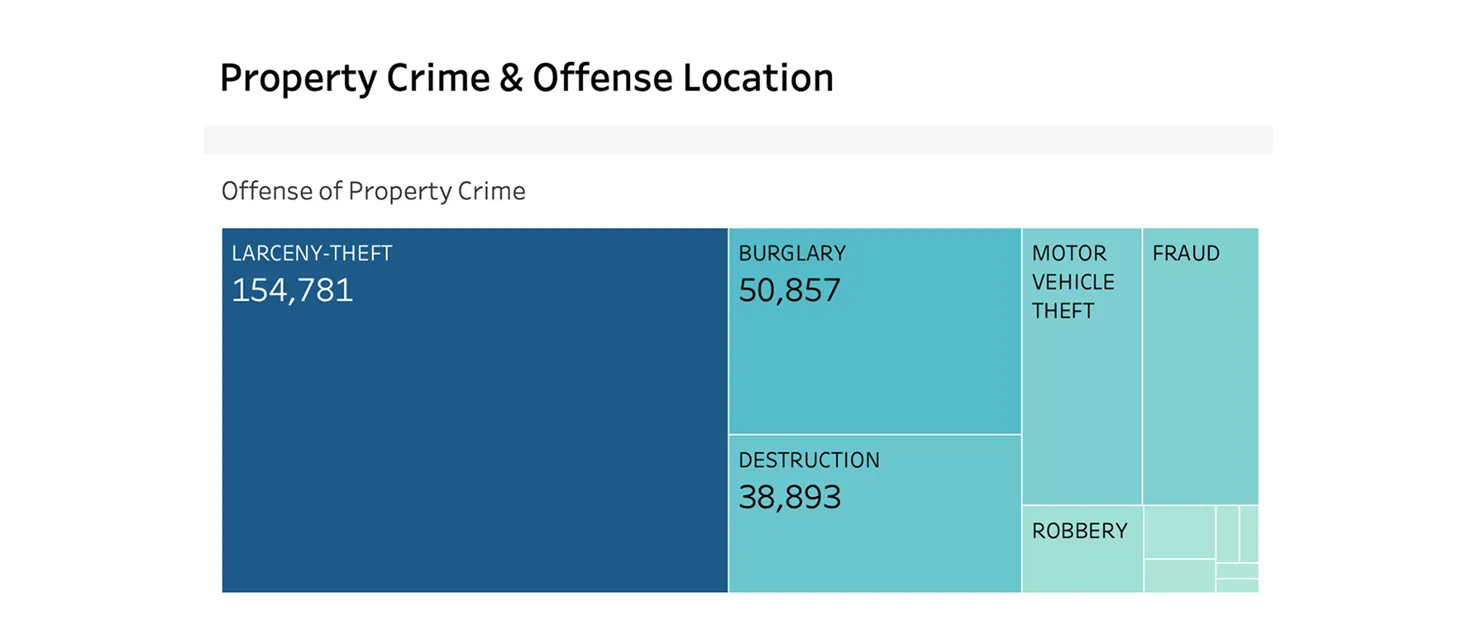
Hotspot Identified: The Downtown Commercial area reports 20,485 property crimes.

The highest across neighborhoods — confirmed by the heatmap, which centers on this district.

Insight 2: When Does Crime Peak?
HYPOTHESIS 2: The occurrence of larceny theft is significantly higher from 4pm-12am.
Reality: Confirmed.
Quarterly & monthly trends show crime spikes in Q4, especially in October.
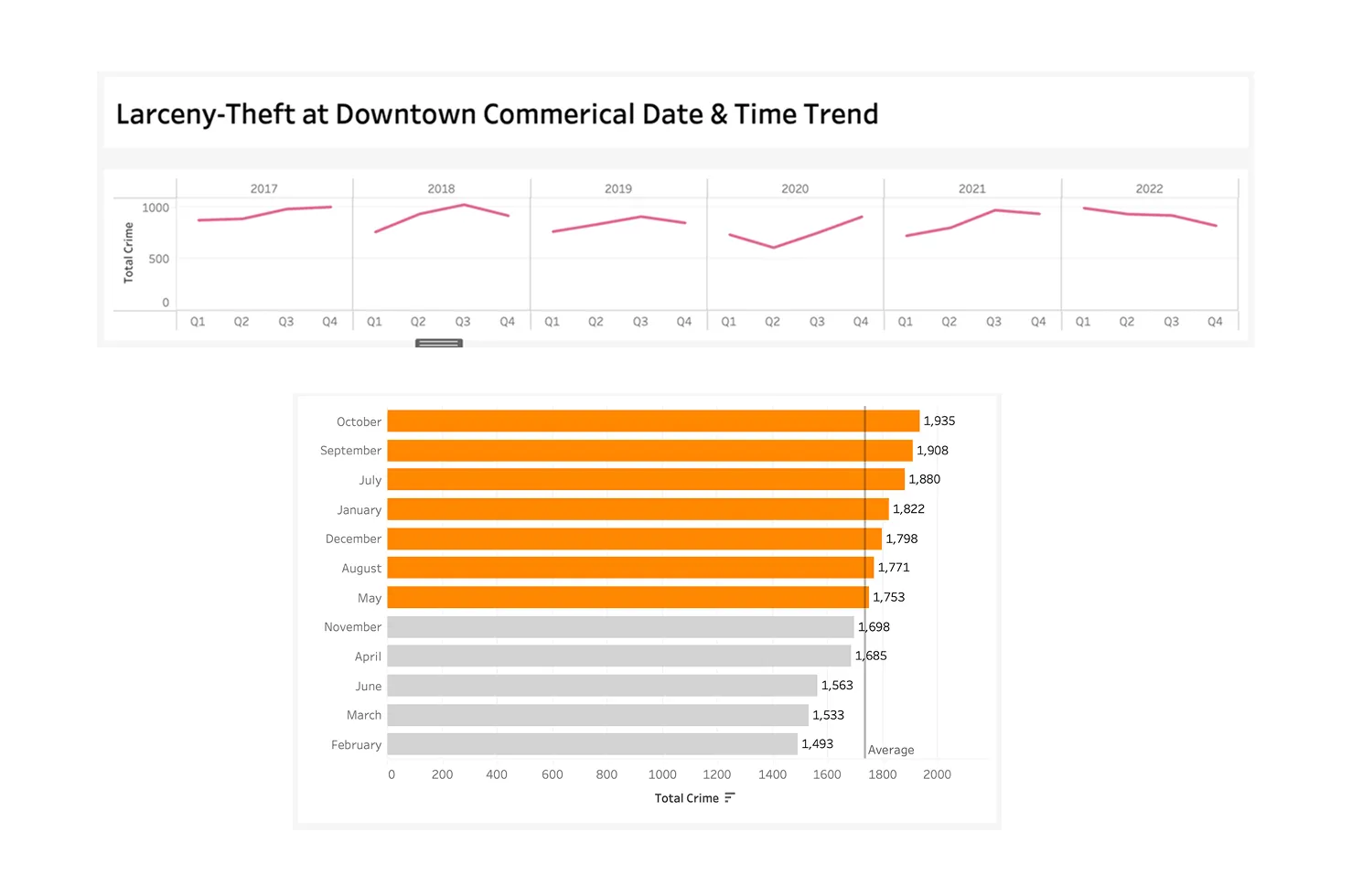
The Day & Time heatmap reveals peak incidents at 12:00 am on From Friday to Sunday, with clear risk windows from 4pm–12am.

Insight 3: Who’s Being Arrested?
Hypothesis: Property crime is most prevalent among men aged 36–45.
Reality: Arrests rose sharply from 20 in 2017 to 5,954 in 2022, reflecting an overall surge in property crime enforcement.

The age range with the most arrests is 26–35, not 36–45, with men leading at 6,159 incidents.
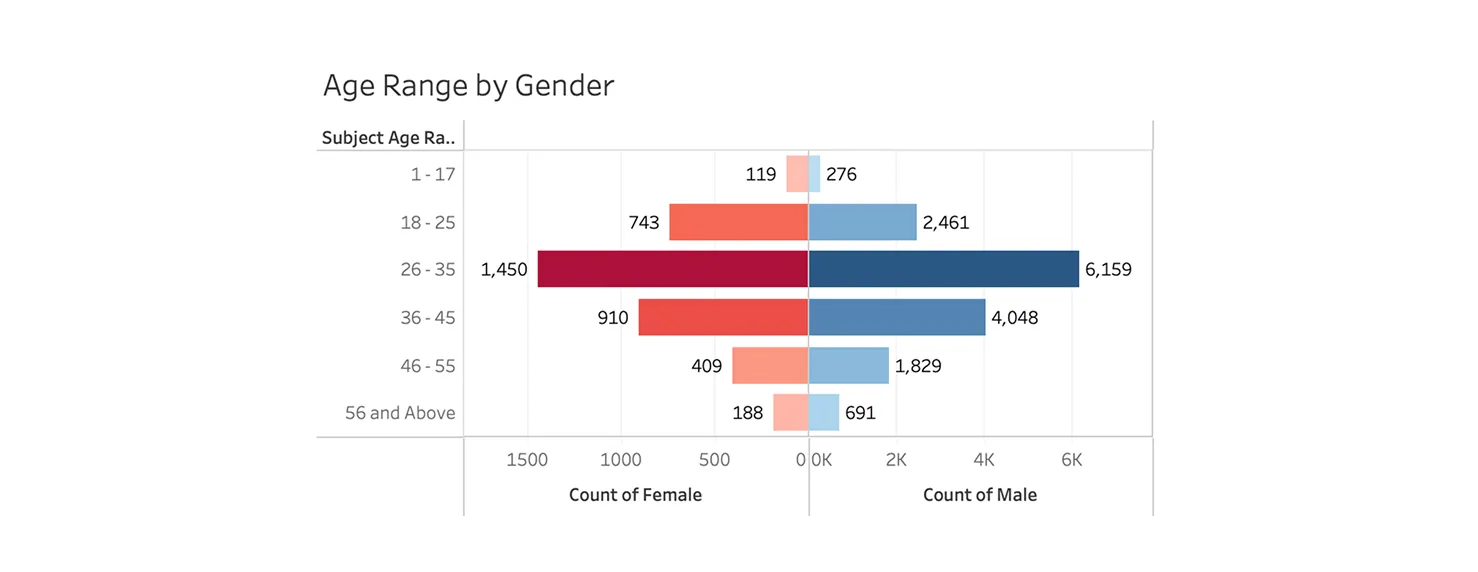
Demographic Breakdown: A race-based treemap reveals that White individuals account for the highest number of arrests (10,205) for property crimes between 2017 and 2022.
